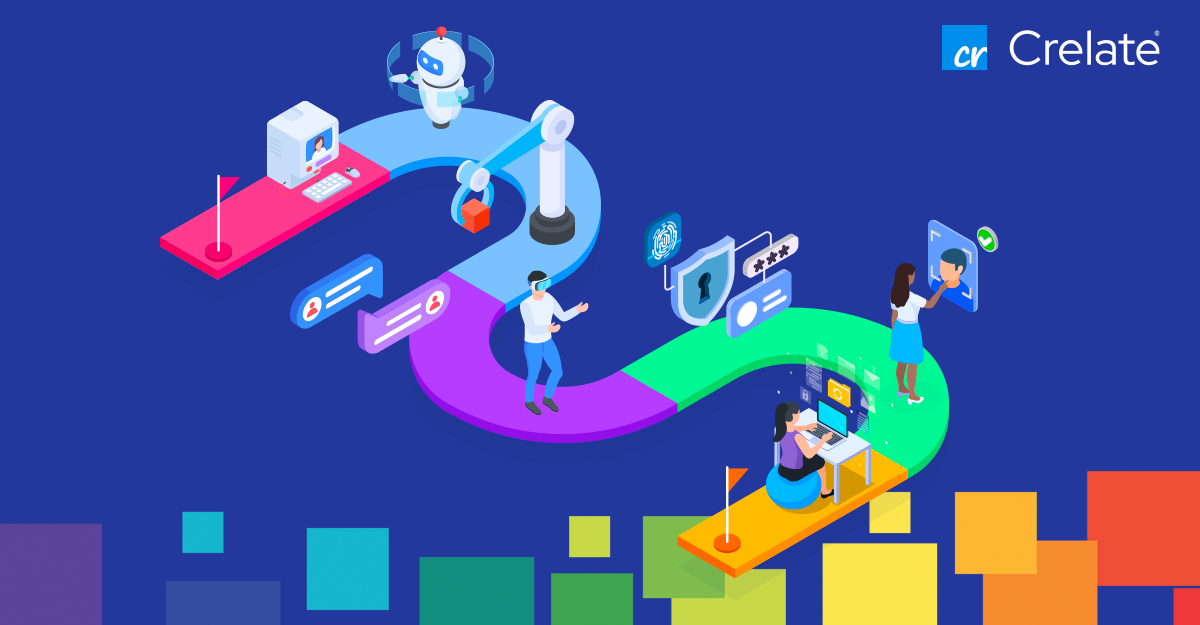
Artificial intelligence has exploded into the mainstream over the past year, with tools like OpenAI’s ChatGPT showcasing the remarkable capabilities of the most recent iterations of proprietary large language models. However, as impressive as these AI models are, they have limitations when it comes to real-world business applications like recruiting.
What AI Is… and What It Isn’t.
I am reminded of Arthur C. Clarke’s famous quote: “Any sufficiently advanced technology is indistinguishable from magic.” Put another way, don’t get swept up in the AI hype without understanding its true nature.
While ChatGPT and other large language models (LLMs) look amazing, it’s important to understand that they’re not knowledge models. They’re language models. They don’t actually know anything, other than the fact that certain words, and patterns, are correlated with other words and patterns. From there you get an output that is put together with a series of prompts (hints and instructions) and made random by a “temperature” setting, which effectively controls the randomness or “creativity” of those connections.
LLMs are a type of artificial intelligence that can recognize and generate text. Since LLMs aren’t knowledge models, they must be trained to be useful. Without getting too technical, they are trained by being given many, many examples and vast amounts of data. The data is turned into numbers or vectors, and stored in a way that the related numbers are closer to each other, and unrelated are further away.. From there, users can issue prompts to the LLM, the prompt is turned into vectors as well and then the magic beings. LLMs make connections on that data, these connections are based on the distance between words, the connections between words, and then the machine can “infer” meaning based on the patterns recognized within that data.
In short, you take a bunch of data, get it processed and interconnected, sometimes in ways a human wouldn’t have seen, and out of that comes a model. And I should add, there are lots of models. There are hundreds of thousands of models, of varying quality and for various purposes available on various public platforms.
Most LLMs are trained on information from the internet and other large corpus of public (and sometimes questionably public) data. As such, these models are only as good as the information they’re fed, the decisions made on how and what to feed them, and the prompts that sit between your asks and the underlying model. In addition, they are only as up to date as of the last time that information was given.
These models have no true understanding, only statistical patterns based on the training data they ingest, which can be outdated. When a model does something unexpected, it’s called a hallucination, which is a cute way of personifying an impersonal problem. Applying such models directly to business problems can certainly have challenges and unexpected results.
Overcoming AI’s Hurdles
For AI to truly revolutionize an industry like recruiting, several key hurdles must be overcome:
- Data Separation: Finding ways to isolate and leverage a company’s proprietary data with AI models.
- Performance and Cost: Developing scalable, cost-effective AI solutions.
- Compliance and Legality: Ensuring AI tools are applied ethically, and comply with rapidly evolving regulations around bias, privacy, and fair hiring practices.
As I look at the landscape of regulation coming down around AI, the advice is this: Don’t use AI for the actual hiring decisions. Don’t trust that to a machine and be careful of where AI is introducing biases or challenges. Be prepared to defend, perhaps in court, the decisions that you do defer to machines. Let this test guide your approach.
AI’s Bias and Other Issues
Regulations around AI bias, privacy, and ethical use of this technology are rapidly evolving. In New York City, there is now an annual audit requirement for any AI system being used for hiring or promotion decisions. Other jurisdictions are looking at similar laws. Even the AI models themselves can inadvertently bake in biases from their training data.
There are also Intellectual Property, or IP, concerns – for example, ChatGPT states that, “As between you and OpenAI, and to the extent permitted by applicable law, you (a) retain your ownership rights in Input and (b) own the Output. We hereby assign to you all our right, title, and interest, if any, in and to Output.” Simply put, this means by using their model you have permission to leverage the information in their output. But do you truly have those rights if that output contains copyrighted data the model ingested during training? Not to mention, you likely don’t even have permission from your candidates to use their data in the AI app you’re using.
Congress is currently considering several bills aimed at reining in potential negative impacts of AI as well. The landscape is shifting quickly. We’ll update this article as new regulations are released but know it’s important to do your research before implementing AI into your processes.
Focus on Productivity to Get to Conversations Faster
AI’s true value in recruiting lies beyond augmenting and accelerating processes around understanding candidate skills and experiences from resumes. AI only knows what the resume explicitly states, and what it can infer by what it states. The resume of course is only a portion of a candidate’s full story; and those inferences could be flawed. Don’t get me started on AI produced resumes, then being read by AI filters. The connections and inference may be useful though and surfacing them so recruiters can make decisions is a great area to explore.
The goal should be to use AI to surface connections and recognize patterns that may not have otherwise been surfaced, and then to get the people connected as efficiently as possible. If you like Candidate A, the machine may let you know about Candidate B, whose resume doesn’t just “look like” A’s, but several steps removed, is similar. It can also be to generate net new content and save some repetitive steps.
However, it is so important to keep the human, individualized element as the crucial centerpiece when it comes to evaluation and hiring decisions. But for accelerating your processes, AI makes sense. Think of it as a personal assistant, not a replacement for your lead recruiter.
Race to the bottom – Keep the Human Element in the Talent Business
If you are looking to use AI to create massive amounts of outreach emails, sequences, texts, you’re late to the party. AI and other tools are just making this easier and more “personalized” to the point of exhaustion and annoyance of recipients. Open rates and response rates are dropping, and some think volume and other AI tricks is the answer. I don’t think it is.
Rather than replacing human recruiters with AI entirely, the real opportunity lies in using AI to accelerate and empower existing recruiting processes. AI should solve for the scenario of accelerating the processes you have today and empowering your recruiters to continue to grow and continue to help people find their next opportunity. The magic is as it has always been, being persistent and different to stand out. Recruiters at the end of the day have to “make a market;” which is hard. And spamming with ever more personalized emails isn’t going to make you stand out.
By overcoming AI’s key hurdles in responsible and compliant ways, Crelate aims to augment recruiters’ abilities, not replace them. AI may be hyped, but bridging its capabilities with human expertise is where the true power lies in the talent industry. Stay tuned for what we’ve been working on…